
When entropy increases, a certain amount of energy becomes permanently unavailable to do work. Entropy is associated with the unavailability of energy to do work. In the second case, entropy is greater and less work is produced. The same heat transfer into two perfect engines produces different work outputs, because the entropy change differs in the two cases. There is 933 J less work from the same heat transfer in the second process. We noted that for a Carnot cycle, and hence for any reversible processes, We can see how entropy is defined by recalling our discussion of the Carnot engine. That unavailable energy is of interest in thermodynamics, because the field of thermodynamics arose from efforts to convert heat to work. Although all forms of energy are interconvertible, and all can be used to do work, it is not always possible, even in principle, to convert the entire available energy into work. Entropy is a measure of how much energy is not available to do work. Recall that the simple definition of energy is the ability to do work. If you liked this post, please follow my blog on Twitter!.Making Connections: Entropy, Energy, and Work That’s all for now! Information gain is just one of many possible feature importance methods, and I’ll have more articles in the future to explore other possibilities. Info$colors <- c("green", "red", "yellow", "green", "yellow", "yellow") Info <- ame(fruits = c("watermelon", "apple", "banana", "grape", "grapefruit", "lemon")) So, how do we calculate information gain in R? Thankfully, this is fairly simple to do using the FSelector package:
How to calculate entropy how to#
= 0.650022 How to calculate information gain in R Here, 5 / 6 of the fruits are round and 1 / 6 is thin. In this case, 3 / 6 of the fruits are medium-sized, 2 / 6 are small, 1 / 6 is big. Likewise, we want to get the information gain for the size variable. Using the formula from above, we can calculate it like this: Proportionally, the probability of a yellow fruit is 3 / 6 = 0.5 2 / 6 = 0.333. 3 out of the 6 records are yellow, 2 are green, and 1 is red. Suppose we want to calculate the information gained if we select the color variable. This is based off the size, color, and shape variables. In the below mini-dataset, the label we’re trying to predict is the type of fruit. Information gain in the context of decision trees is the reduction in entropy when splitting on variable X. In this way, entropy can be thought of as the average number of bits needed to encode a value for a specific variable. A microstate (W) is a specific configuration of the locations and energies of the atoms or molecules that comprise a system. However, it’s common to use base 2 because this returns a result in terms of bits. Following the work of Carnot and Clausius, Ludwig Boltzmann developed a molecular-scale statistical model that related the entropy of a system to the number of microstates possible for the system. Why the log 2? Technically, entropy can be calculated using a logarithm of a different base (e.g. The closer you get to a variable having a single possible value, the less information that single value gives you.
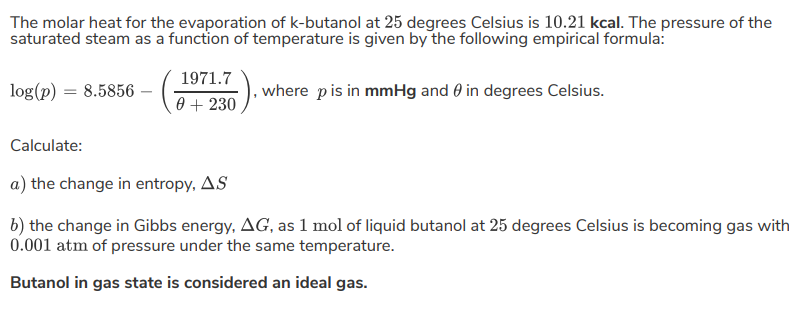
This means that the variable provides no real information about the data.
How to calculate entropy movie#
Well – that predictor is useless! Mathematically speaking, it’s useless because every record in the dataset is a movie – so there’s a 100% probability of that event (i.e. One of your predictors is a binary indicator – 1 if the record refers to a movie, and 0 otherwise. Suppose you’re predicting how much a movie will make in revenue. That may sound a little abstract at first, so let’s consider a specific example. In other words, the amount of information about an event (or value) of X decreases as the probability of that event increases.

Also, entropy as a function is monotonically decreasing in the probability, p. The formula has several useful properties. Why is it calculated this way?įirst, let’s build some intuition behind the entropy formula. p(x i) is the probability of a particular (the i th) possible value of X. Here, each X i represents each possible (i th) value of X. How to calculate entropyĮntropy of a random variable X is given by the following formula: Entropy measures the amount of information or uncertainty in a variable’s possible values.
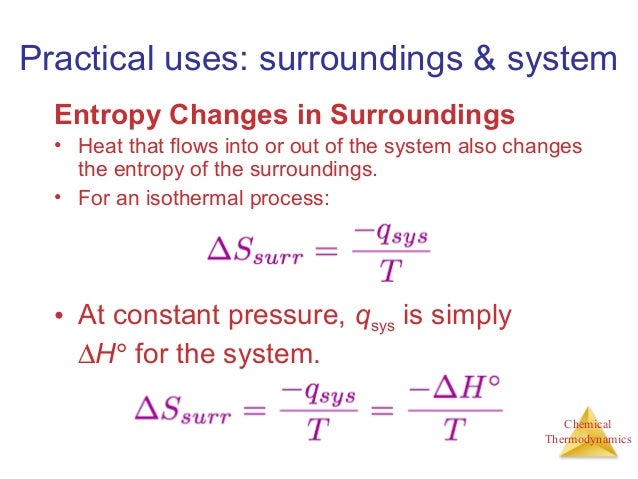
Before we formally define this measure we need to first understand the concept of entropy. Information gain is a measure frequently used in decision trees to determine which variable to split the input dataset on at each step in the tree. We’ll start with the base intuition behind information gain, but then explain why it has the calculation that it does. This post will explore the mathematics behind information gain.
